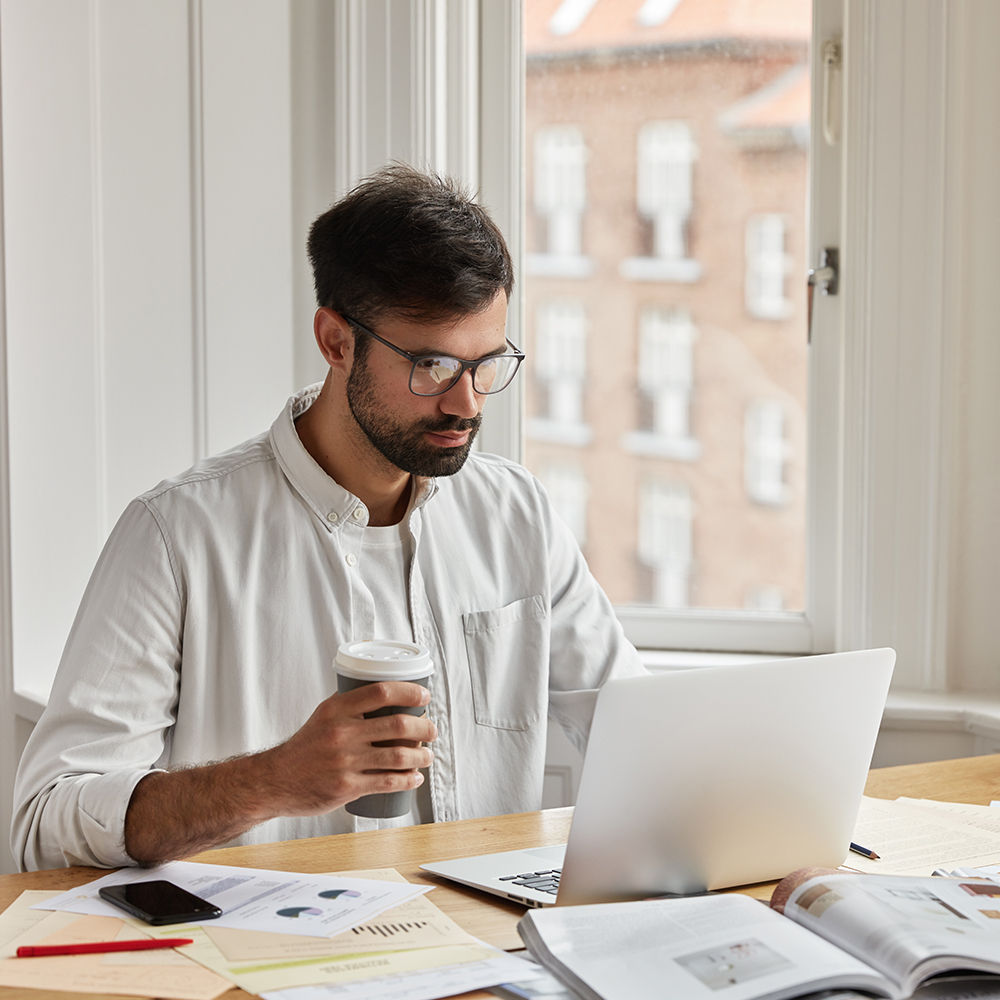
- January 27, 2022
- Latest News
- Pedropiri
- 3 Comments
The Big Picture…
Innovation spreads through specific pathways within a social network.
But could our understanding of network structure help us predict—or even accelerate—this diffusion?
Identifying Key Influencers
By mapping out who holds the most sway in a network, we can target efforts for the greatest ripple effect.
The Idea
When examining how innovations permeate a community, we invoke the standard definition:
“Diffusion is the process by which an innovation is communicated through certain channels over time among the members of a social system.”
— Rogers, Diffusion of Innovations
I approached this from a graph-theory standpoint. First, building on Bass’s distinction between innovators and imitators; then expanding to Rogers’s five adopter categories. Visually, each adopter group can be represented as clusters on a network diagram, with connections propagating from the earliest adopters outward over time.
Although the schematic we used wasn’t perfectly accurate, it served to clarify the process. Diffusion propagates through the nodes of a network via specific pathways over time. It begins with Innovators, moves on to Early Adopters, then the Early Majority, and so on. We’ve observed a “chasm” between the Early Adopters and the Early Majority—especially pronounced in high-tech markets—and Maloney taught us that the messaging and channels effective for that first 16% must differ from those used for the remaining segments.
Since diffusion unfolds over a network, can principles of network topology help us predict whether its layout will aid information flow or present obstacles? And how might we leverage the network’s structure to our advantage? As Pyo, Gruca, and Russell demonstrate:
“A non-adopter adopts more rapidly the higher the fraction of her friends who are adopters.”
— A New Bass Model Utilizing Social Network Data
Network topology offers the promise of identifying key influencers, loosely knit adopter clusters, mavens, and potential bottlenecks. But what exactly is network topology?
Network Topology
Networks can take many forms. In some cases, they exhibit a hub-and-spoke configuration, where dense clusters of nodes link to one another through a handful of central connectors.
(Figure X)
Others, the network may take on a volcano shape, with nearly every node linked directly to a single central hub. In contrast, some networks form a toroidal structure, where connections wrap around in a continuous loop.
If we can map out the social system’s topology, we gain foresight into how diffusion will progress, where it might stall, and what levers we can pull to boost adoption. Although we often sketch these patterns visually, it’s more precise to define them by a set of measurable network attributes.
Network attributes
Those colloquial topology labels correspond to specific configurations of core network metrics—examples of which are summarized in Figure 3.
We could write article after article on network topologies and metrics. You can start with this Wikipedia overview or dive into this detailed Semantic Scholar paper.
What Do Attributes Tell Us About Diffusion?
Network attributes codify visual topologies into measurable traits. By quantifying these, we can forecast whether an innovation will spread smoothly or hit roadblocks—and identify levers to pull for better reach.
Let’s look at some concrete examples:
Density and Diffusion Success Probability
- High density: Imagine 100 people where each is connected to all 99 others. Density = 99/99 = 100%. Plenty of overlapping pathways ensure diffusion persists even if some links fail.
- Low density: Now suppose each person can directly reach only 2 neighbors. Density ≈ 2/99 = 2%. Here, a single non-participating individual can sever the chain and stop diffusion entirely.
Weak Ties – Crossing Group Boundaries
Picture several clusters, each defined by a shared attribute. A handful of weak ties loosely link one group to the next, enabling ideas to hop between clusters. But if those bridge-nodes don’t pass along the innovation, a “chasm” emerges and diffusion stalls.
Structural Holes – Carrying Innovation
Think of IT consultancies: they operate across diverse industries, effectively spanning structural holes. This unique position allows them to “carry” innovations from one sector and introduce them into another.
Eigenvectors and Mavens
Eigenvector centrality ranks nodes by the influence of their neighbors—Google’s original PageRank did exactly this. High-eigenvector nodes often correspond to the mavens Malcolm Gladwell describes in The Tipping Point.
Degree Centrality and Social Influencers
Individuals with exceptionally high out-degree (many direct connections) tend to be social influencers—prime candidates for seeding and accelerating diffusion.
Finding a Network’s Topology
In principle, mapping these attributes reveals influencers, weak points, and optimal diffusion pathways. In practice, uncovering the true social graph is more complex:
- Within organizations: The formal org chart seldom reflects actual communication patterns. Surveys or analysis of email threads and collaboration tools can expose the real topology.
- Beyond organizations: People resist surveys, and private communications are inaccessible. However, public platforms like LinkedIn and Facebook offer partial network views.
For tools that aid this analysis, see resources on social network analysis software.
“It is now clear that different types of network structures yield predictable differences in aggregate diffusion curves.”
— Dover, Goldenberg & Shapira (2012); Trusov, Rand & Joshi (2013)
Source: A New Bass Model Utilizing Social Network Data (Pyo, Gruca & Russell)
https://www.researchgate.net/…/A-New-Bass-Model-Utilizing-Social-Network-Data.pdf?origin=publication_detail
Research shows that calibrating the classical Bass model on sparsely connected networks skews parameter estimates:
- Social influence (q) is underestimated.
- External influence (p) is overestimated.
In contrast, the Network-Based Bass (NBB) model yields accurate diffusion parameters regardless of topology—and outperforms the traditional Bass fit.
Pyo, Gruca & Russell, “A New Bass Model Utilizing Social Network Data”
https://www.researchgate.net/…/A-New-Bass-Model-Utilizing-Social-Network-Data.pdf?origin=publication_detail
3 Replies to “Innovation: Diffusion and the Insights of Network Topology”
Mahfuza
Lorem ipsum dolor sit amet, consectetur adipiscing elit. Sed cursus sol- licitudin odio, eu tempor velit pellentesque et Sed ut perspiciatis
Asfia
Lorem ipsum dolor sit amet, consectetur adipiscing elit. Sed cursus sollicitudin odio, eu tempor velit et Sedtis
Rashida
Lorem ipsum dolor sit amet, consectetur adipiscing elit. Sed cursus sol- licitudin odio, eu tempor velit pellentesque et Sed ut perspiciatis